New SFB 1313 publication (University of Stuttgart), published in Water Resources Research. The paper has been prepared within SFB 1313's research project B04.
Authors
- Sebastian Reuschen (University of Stuttgart, SFB 1313 research project B04)
- Fabian Jobst
- Wolfgang Nowak (University of Stuttgart, SFB 1313 research projects B04 and B05)
Abstract
In geostatistics, Gaussian random fields are often used to model heterogeneities of soil or subsurface parameters. To give spatial approximations of these random fields, they are discretized. Then, different techniques of geostatistical inversion are used to condition them on measurement data. Among these techniques, Markov chain Monte Carlo (MCMC) techniques stand out, because they yield asymptotically unbiased conditional realizations. However, standard Markov Chain Monte Carlo (MCMC) methods suffer the curse of dimensionality when refining the discretization. This means that their efficiency decreases rapidly with an increasing number of discretization cells. Several MCMC approaches have been developed such that the MCMC efficiency does not depend on the discretization of the random field. The pre-conditioned Crank Nicolson Markov Chain Monte Carlo (pCN-MCMC) and the sequential Gibbs (or block-Gibbs) sampling are two examples. This paper presents a combination of both approaches with the goal to further reduce the computational costs. Our algorithm, the sequential pCN-MCMC, will depend on two tuning-parameters: the correlation parameter of the pCN approach and the block size
of the sequential Gibbs approach. The original pCN-MCMC and the Gibbs sampling algorithm are special cases of our method. We present an algorithm that automatically finds the best tuning-parameter combination (
and
) during the burn-in-phase of the algorithm, thus choosing the best possible hybrid between the two methods. In our test cases, we achieve a speedup factors of
over pCN and of
over Gibbs. Furthermore, we provide the MATLAB implementation of our method as open-source code.
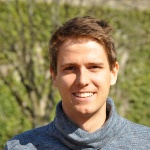
Sebastian Reuschen
M.Sc.Doctoral Researcher, Research Project B04
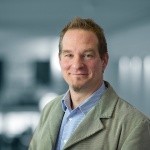
Wolfgang Nowak
Prof. Dr.-Ing.Principal Investigator, Research Projects B04 and B05