People
Sebastian Reuschen (Alumnus)
Claus Haslauer (Alumnus)
Dirk Schlabing (Alumnus)
Publications
Preprints
- Scheurer, S., Reiser, P., Brünnette, T., Nowak, W., Guthke, A., & Bürkner, P.-C. (2025). Uncertainty-Aware Surrogate-based Amortized Bayesian Inference for Computationally Expensive Models. In arXiv preprint arXiv:2505.08683. https://doi.org/10.48550/arXiv.2505.08683
Conference Papers
- Brünnette, T., & Nowak, W. (2024). Efficient Inference for non-deterministic fractures. In J. Gómez-Hernández, E. Varouchakis, D. Hristopulos, G. Karatzas, P. Renard, & M. J. a. Pereira (Eds.), geoENV2024 Book of Abstracts (pp. 67–68). https://2024.geoenvia.org/wp-content/uploads/sites/8/2024/07/BookOfAbstracts_OnlyAbstracts_v3_corr.pdf
Journal Articles
- Kröker, I., Brünnette, T., Wildt, N., Morales Oreamuno, M. F., Kohlhaas, R., Oladyshkin, S., & Nowak, W. (2025). Bayesian3 active learning for regularized multi-resolution arbitrary polynomial chaos using information theory. International Journal for Uncertainty Quantification, 15, Article 3. https://doi.org/10.1615/Int.J.UncertaintyQuantification.2024052675
- Nowak, W., Brünnette, T., Schalkers, M., & Möller, M. (2024). Overdispersion in gate tomography: Experiments and continuous, two-scale random walk model on the Bloch sphere. ACM Transactions on Quantum Computing, 5, 1–17. https://doi.org/10.1145/3688857
- Hermann, F., Michalowski, A., Brünnette, T., Reimann, P., Vogt, S., & Graf, T. (2023). Data-Driven prediction and uncertainty quantification of process parameters for directed energy deposition. Materials, 16, Article 23. https://doi.org/10.3390/ma16237308
- Wagner, A., Sonntag, A., Reuschen, S., Nowak, W., & Ehlers, W. (2023). Hydraulically induced fracturing in heterogeneous porous media using a TPM-phase-field model and geostatistics. Proceedings in Applied Mathematics and Mechanics, 23. https://doi.org/10.1002/pamm.202200118
- Cheng, K., Lu, Z., Xiao, S., Oladyshkin, S., & Nowak, W. (2022). Mixed covariance function Kriging model for uncertainty quantification. International Journal for Uncertainty Quantification, 12, Article 3. https://doi.org/10.1615/Int.J.UncertaintyQuantification.2021035851
- Gonzalez‑Nicolas, A., Bilgic, D., Kröker, I., Mayar, A., Trevisan, L., Steeb, H., Wieprecht, S., & Nowak, W. (2022). Optimal exposure time in gamma-ray attenuation experiments for monitoring time-dependent densities. Transport in Porous Media, 143, 463–496. https://doi.org/10.1007/s11242-022-01777-5
- Chow, R., Parker, B., Steelman, C., Thoms, A., & Nowak, W. (2021). How do fractures influence hyporheic exchange in sedimentary rock riverbeds? Water Resources Research, 57. https://doi.org/10.1029/2020WR028476
- Reuschen, S., Jobst, F., & Nowak, W. (2021). Efficient discretization-independent Bayesian inversion of high-dimensional multi-Gaussian priors using a hybrid MCMC. Water Resources Research, 57. https://doi.org/10.1029/2021wr030051
- Reuschen, S., Nowak, W., & Guthke, A. (2021). The four ways to consider measurement noise in Bayesian model selection - and which one to choose. Water Resources Research, 57. https://doi.org/10.1029/2021WR030391
- Xiao, S., Xu, T., Reuschen, S., Nowak, W., & Hendricks Franssen, H.-J. (2021). Bayesian Inversion of multi-Gaussian log-conductivity fields with uncertain hyperparameters: An extension of preconditioned Crank-Nicolson Markov Chain Monte Carlo with parallel tempering. Water Resources Research, 57. https://doi.org/10.1029/2021WR030313
- Höge, M., Guthke, A., & Nowak, W. (2020). Bayesian model weighting: The many faces of model averaging. Water, 12. https://doi.org/10.3390/w12020309
- Reuschen, S., Xu, T., & Nowak, W. (2020). Bayesian inversion of hierarchical geostatistical models using a parallel-tempering sequential Gibbs MCMC. Advances in Water Resources, 141. https://doi.org/10.1016/j.advwatres.2020.103614
- Xu, T., Reuschen, S., Nowak, W., & Hendricks Franssen, H.-J. (2020). Preconditioned Crank-Nicolson Markov Chain Monte Carlo coupled with parallel tempering: An efficient method for Bayesian inversion of multi-Gaussian log-hydraulic conductivity fields. Water Resources Research, 56. https://doi.org/10.1029/2020wr027110
- Xiao, S., Reuschen, S., Köse, G., Oladyshkin, S., & Nowak, W. (2019). Estimation of small failure probabilities based on thermodynamic integration and parallel tempering. Mechanical Systems and Signal Processing, 133. https://doi.org/10.1016/j.ymssp.2019.106248
Research
About this Project
Project B04 focuses on random descriptions of irregular fracture geometries, fracture networks, and heterogeneous background matrices. From there, it proceeds to investigate the consequences of irregularity and heterogeneity for relevant processes occurring in such fractured porous media. These are relevant for accurate predictions, reservoir characterization, uncertainty quantification, and probabilistic risk assessment in hydromechanical and geothermal applications.
Results
Funding Period 1
In the first funding phase, we represented fracture networks as elevated hydraulic conductivity values with channel-like structures in (randomly) heterogeneous porous media. Following this approach, we developed efficient Markov Chain Monte Carlo (MCMC) methods for Bayesian inversion of such fields. We also developed a novel statistical analysis and random generation of fracture images via the Radon transform (see Figure 1). The extended-and-randomized Radon transform can generate randomized fracture images that match the statistics of fracture length, aperture (including roughness), density, angles and connectivity, as provided by an example image.
Funding Period 2
In the current (second) funding phase, we investigate the effects of (random) material heterogeneity on the initiation and propagation of fractures. This involves a fundamentally new approach to stochastic modeling of fracture propagation: we incorporate a random series of directional decisions during fracture propagation, resulting in irregular fracture patterns, as seen in Figure 2. We implement this inside the time stepping of a phase field model from Project B01.
We also collaborate with Project B02 on the use of spatial random fields of mechanical (Lamé) parameters. These advances provided insights into the scaling behavior between mechanical heterogeneity and irregular fracture geometries (Figure 3). We are further collaborating with Project D03, Project B03, and Project INF on the stochastic validation of transport models with irregular and uncertain fracture geometries.
Future Work
Our goal for the third funding period will be to understand the effects of heterogeneity and irregularities on processes such as pressure diffusion during hydraulic stimulation and the transfer of heat and mass. Figure 4 exemplifies such a complex domain. Specific goals will include: previously unexplored phenomena during responses of fracture networks to oscillatory hydraulic stimulation, developing simplified proxy models for the effective behavior of pressure diffusion, and investigating consequences on heat/mass transport. We will build on a pressure diffusion simulation framework composed in FEniCS and preCICE from project D02, collaborate on realistic statistics of fracture networks and mechanically heterogeneous materials with projects B02 and B05, and perform benchmarking our proxy models jointly with D03.
Contributing to the “salt vision” of the overall CRC, we will also investigate the effect of clogging in fracture networks on the aforementioned processes, where we discuss the formulation of fracture clogging as a random growth problem with C04.
International Cooperation
Stanford University, USA
We collaborate with Prof. Daniel Tartakovsky at Stanford University to investigate the statistics of fracture initiation in materials under mechanic, thermal or chemically-induced stress, with possible application to battery materials. Prof. Tartakovsky is also the host for a three-month research stay of the project researcher in B04.
TU Delft, The Netherlands
Exploiting similarities between random angles at fracture tips and random angles on the so-called Bloch sphere, we collaborated with Prof. Matthias Möller at TU Delft on the noise behavior of qubits in Noisy intermediate-scale quantum computers.
University of Lausanne, Switzerland
In funding phase one, we exchanged with Prof. Niklas Linde at the University of Lausanne, an international expert on geostatistical and geophysical inversion.
University of Guelph, Canada
We joined forces in the first funding phase with Prof. Beth Parker at the University of Guelph. Together, we modeled the exchange of water and solutes between rivers and riverbeds consisting of fractured rock.
For further information please contact
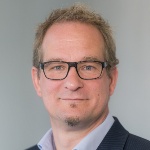
Wolfgang Nowak
Prof. Dr.-Ing.Project Leader, Research Projects B04 and B05