Sebastian Reuschen, doctoral researcher at the Department of Stochastic Simulation and Safety Research for Hydrosystems LS3 of the Institute for Modelling Hydraulic and Environmental Systems, and member within the framework of SFB 1313 and the Integrated Research Training Group IRTG-IMPM, will defend his dissertation:
Title: "Bayesian Inversion and Model Selection of Heterogeneities in Geostatistical Subsurface Modeling"
Date: 2 December 2021
Time: 2 pm CET
Venue: Hybrid event. If you are interested in participating the PhD defense, please contact sebastian.reuschen@iws.uni-stuttgart.de
Abstract
The planning of drinking water supply and the evaluation of subsurface CO2 and nuclear waste storage sites are just a few examples that require knowledge about the subsurface structure. While drinking water wells should be built in regions with highly permeable soils, nuclear waste storage sites should be built in impermeable salt rock. In this example, the hydraulic conductivity can be used to describe the permeability of soils. Determining such subsurface parameters is difficult because they are heterogeneous and only scarce data is available. Consequently, the spatial distribution of subsurface parameters is uncertain. To overcome this challenge, parameter inference and uncertainty quantification can be used to (1) improve predictions and (2) quantify remaining uncertainties in various applications.
This thesis aims to improve the inference and uncertainty quantification of relevant subsurface parameters. To reach this goal, a two-step approach is taken. First, Bayesian inversion, also known as Bayesian inference, is used for probabilistic predictions of subsurface parameter fields. Here, Bayesian inversion defines a so-called posterior distribution which is the updated belief of the prior parameter model given data. Analytical calculation of the posterior is often impossible. Instead, numerical sampling methods can be used to approximate the posterior. Markov chain Monte Carlo (MCMC) methods have shown great potential in doing so. Depending on the amount of available data and type of prior field, MCMC methods still have two drawbacks. In some problem classes, existing MCMC methods are too slow to be used commonly. In other problem classes, no MCMC methods exist to perform Bayesian inversion. This thesis closes these gaps by developing novel MCMC methods to enable or speed up Bayesian inversion. Second, all MCMC methods assume some prior geostatistical models. Selecting appropriate prior geostatistical models can be done using Bayesian model selection (BMS). This thesis shows that BMS results can differ based on where measurement noise is modeled and gives guidance on how to model noise correctly.
Chair: Prof. Dr.-Ing. Holger Steeb (University of Stuttgart)
First Supervisor: Prof. Dr.-Ing. Wolgang Nowak (University of Stuttgart)
Secondary Supervisor: Prof. Dr. rer. nat. Dr.-Ing. habil.András Bárdossy (University of Stuttgart)
Secondary Supervisor: Prof. Dr. Niklas Linde (University of Laussane)
Contact
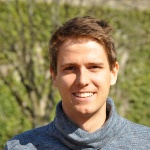
Sebastian Reuschen
Dr.-Ing.Alumnus: Post-doctoral Researcher, Research Project B04